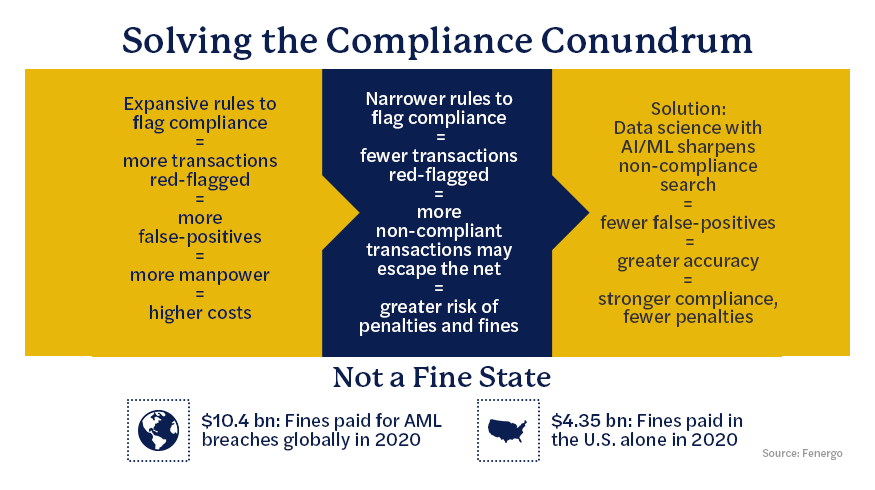
Organizations are implementing new stress testing models to gauge investment risk and meet regulatory compliances — a challenge heightened by the coronavirus pandemic. This has led to increased investments in data sciences and automation solutions, thereby cutting costs incurred due to compliance failures.
There is a classic dichotomy in the area of compliance. On the one hand, a company needs to identify areas of non-compliance. In the process, on the other hand, it might end up finding a plethora of transactions that need to be investigated for non-compliance, and spend most of its energy, time and money in investigating false positives.
As companies install more regulations to identify more potential risks, the number of false positives increases manifold. In order to mitigate this problem, organizations have begun to invest significantly in data sciences services and in machine learning and artificial intelligence. This, they hope, will reduce the number of false positives and enable them to identify non-compliance with greater accuracy.
Companies are investing also in anti-money laundering (AML) solutions, which form a large part of the compliance exercise, and for good reason. Failing to detect fraud or money laundering activities can get companies on the wrong side of regulators. Globally in 2019, fines worth $8.14 billion were levied for AML-related breaches; U.S. regulators alone imposed $2.29 billion of those fines.
Compliance obligations
As part of their AML efforts, companies must collate and analyze data for compliance in areas of trade, employee due diligence and electronic communication surveillance. That involves recording and storing telephone conversations for at least three years, according to FINRA (Financial Industry Regulatory Authority).
This results in the collection of a large volume of data that needs to be analyzed. Companies must frame rules that will help establish areas of non-compliance. That exercise includes identifying anomalies that might warrant further investigation.
For instance, if an anomaly is detected in a phone call and is red-flagged by the system, the company needs to assign an expert to investigate that. For large multinational banks, trying to identify anomalies can be a monumental exercise. Large amounts of money are spent on investigating false positives; many organizations have set up teams in countries where labor is cheap to conduct those investigations.
For instance, a money transfer to Iran will be red-flagged as it is considered a high-risk country. But that money might be a contribution to the Red Cross, which may use it for humanitarian work in that country. This is a classic case of false positive, as Red Cross donations do not fall within the ambit of the controls in place. Normally, an expert would understand this and override the control to eliminate the false positive. Now machine learning and artificial intelligence can take over the role of eliminating false positives by assimilating the skills of overriding those.
Key takeaways
- Organizations have begun to invest significantly in data science in order to reduce false positives in regulatory compliance tracking.
- Machine learning and artificial intelligence can take over the role of eliminating false positives.
- Companies must frame rules that will help establish areas of non-compliance.
- Investments in AML solutions have significantly increased as the failure to detect fraud or money laundering activities can get them on the wrong side of regulators.
Contact
Our experts can help you find the right solutions to meet your needs.
Get in touch