Home » Services » Data & Analytics » Delivering intelligence with speed and scale
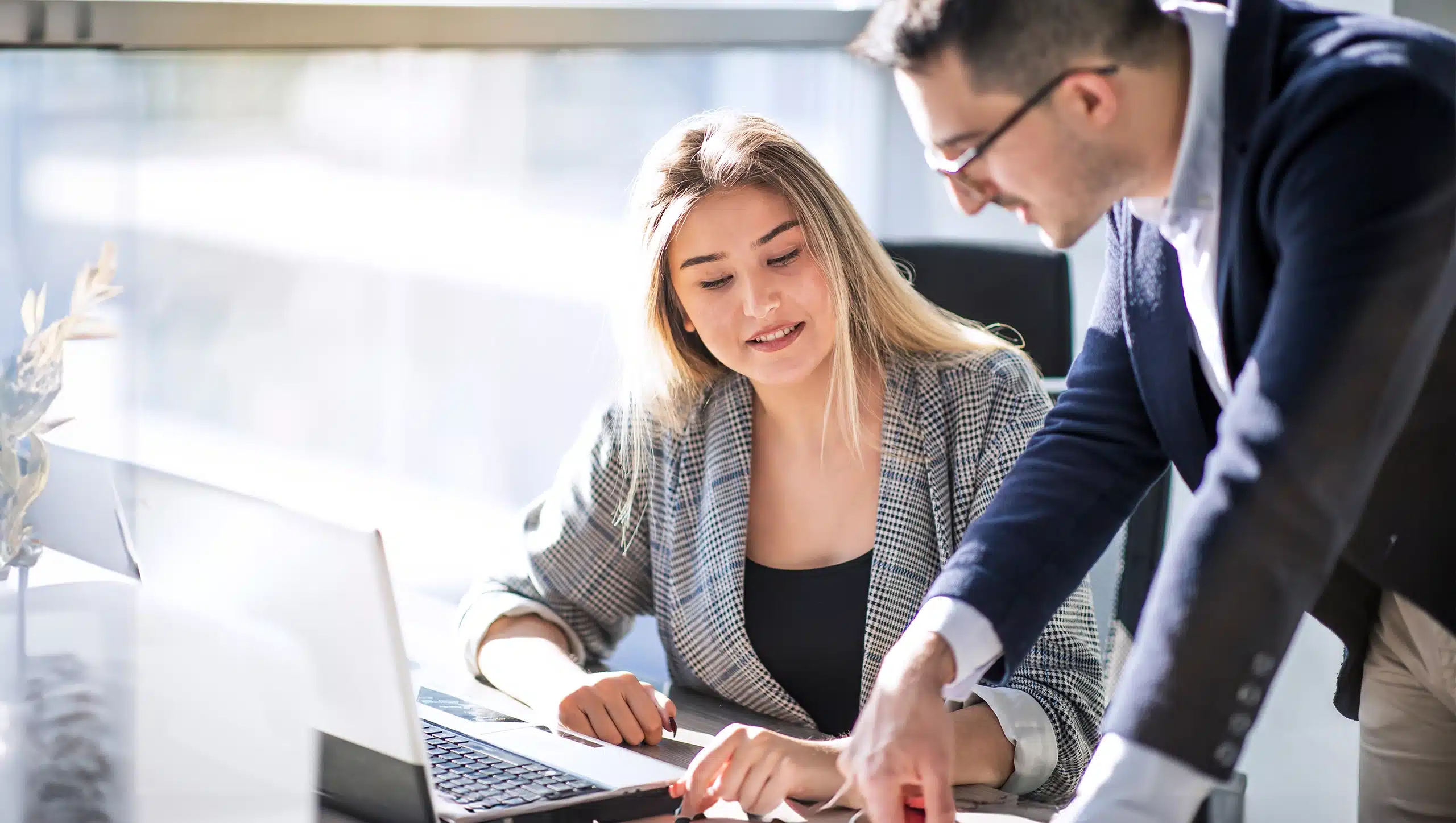
Delivering intelligence with speed and scale
Data Science Engineering and Data & ML Ops are key to enable scaling of the intelligence part of the data monetization lifecycle in cloud.

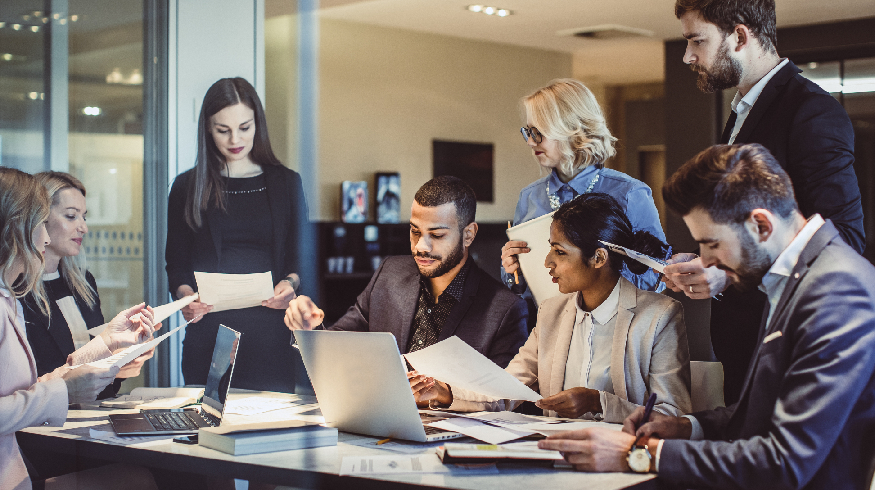
Do you trust your data?
Data driven organizations are ensuring that their Data assets are cataloged and a lineage is established to fully derive value out of their data assets.

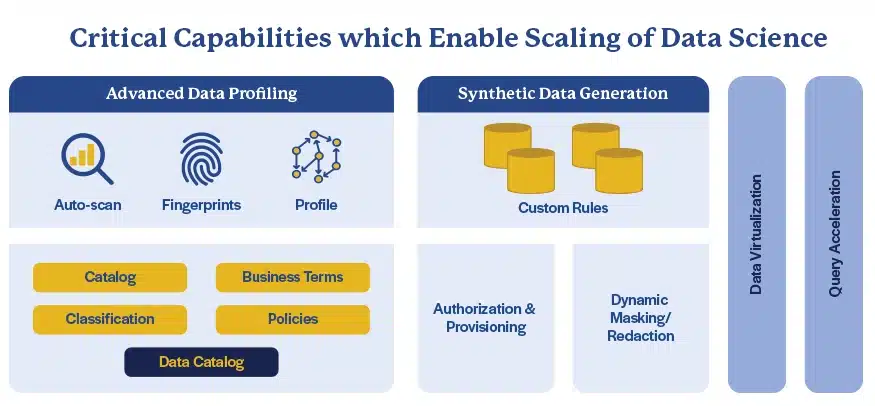
Through a number of digital initiatives over the past decade, organizations have collated a lot of information. In addition to structured data, they are collating unstructured and semi-structured formats, e.g., digitized contracts and audio/video of customer interactions. The opportunities to apply established and emerging AI/ML techniques and models to this wide variety of information and derive intelligence and enhanced insights have significantly increased.
Cloud and the evolving technologies around Data Engineering, Data & ML Ops, Data Science, and AI/ML (e.g., Generative AI) offer a significant opportunity to overcome the limitations and deliver intelligence with speed and at scale. While the number and sophistication of AI/ML models available have increased and become easier to deploy, train/tune, and use, they need information at scale to be transformed to features. Delivering intelligence in scale would require more than just data lakes and lake-houses. It also requires the overall ability to support multiple modeling/data science teams working on multiple problems/opportunities concurrently. Data Science Engineering and Data & ML Ops are key to enable scaling of the intelligence part of the data monetization lifecycle. Teams need to understand data science/modeling lifecycles to effectively scale intelligence.
In conclusion, organizations demand intelligence in scale and at speed. Emerging technologies like Generative AI demand more powerful infrastructure (e.g., GPU farms). Cloud technologies and services enable these. With support for Python across the intelligence lifecycle, it has become easier to bring together data engineering and data science teams that are easier to provision and use in cloud.
To know more about the benefits, challenges, and best practices for scaling various stages of deriving intelligence from data on cloud environments, read the perspective paper here.
Contact
Our experts can help you find the right solutions to meet your needs.
Get in touch