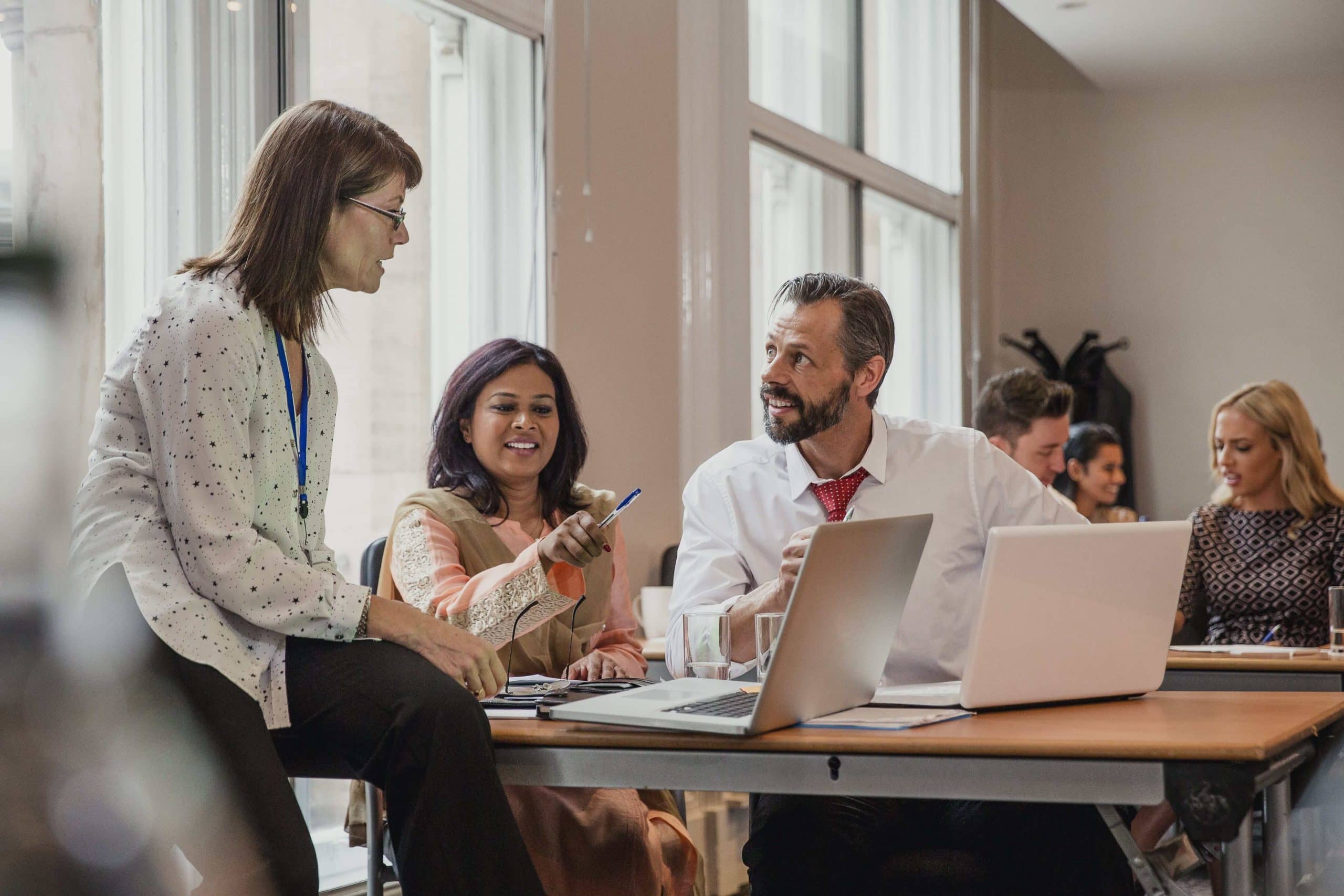
Data and Analytics
Using Data Science to cut BI costs
A proven strategy for report rationalization.

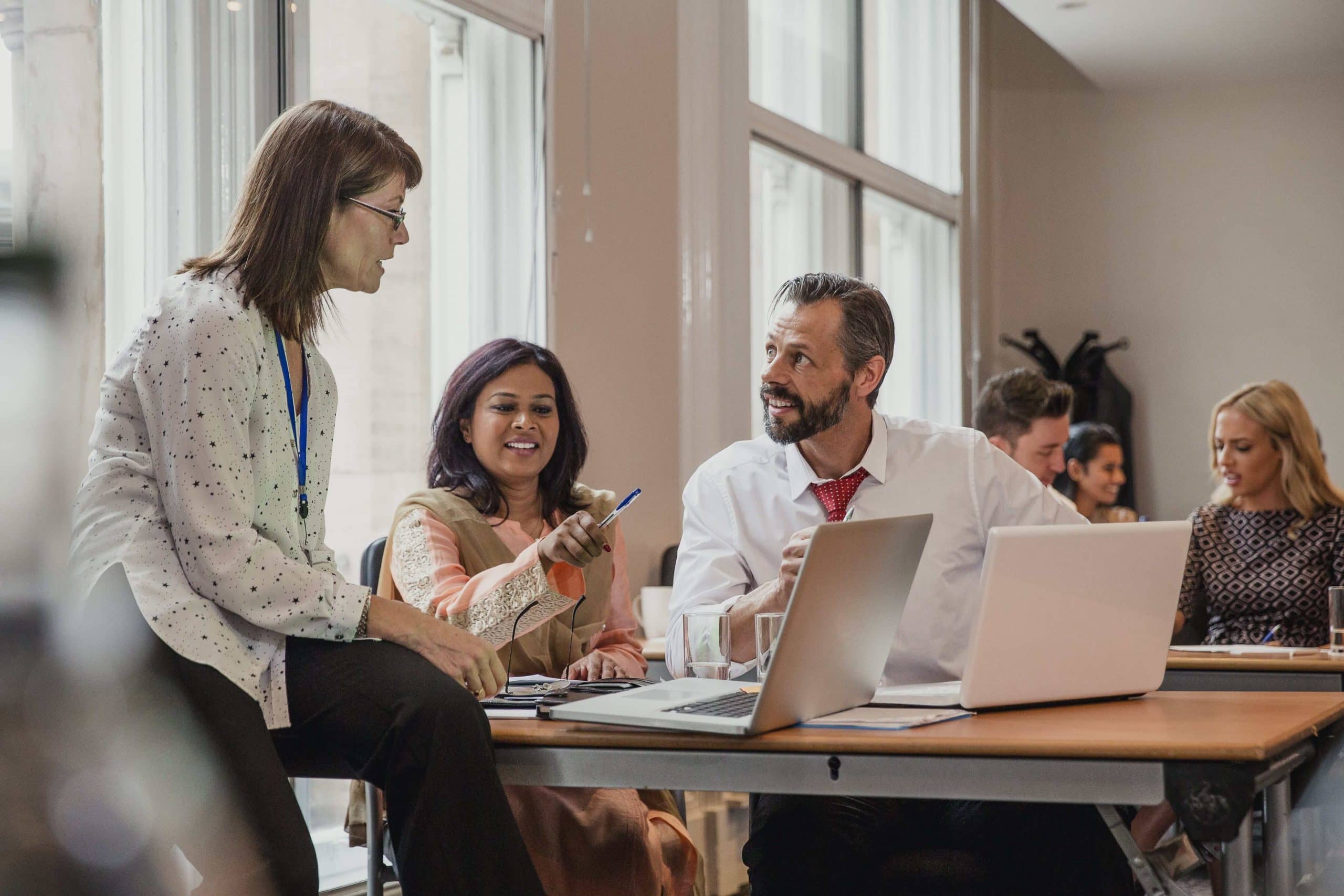
Data and Analytics
Using Data Science to cut BI costs
A proven strategy for report rationalization.

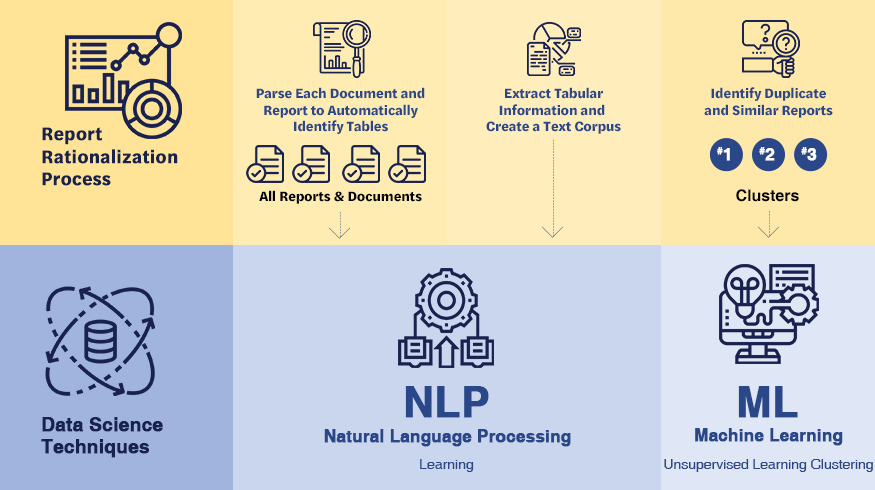
Organizations today rely more than ever on accurate and efficient reporting to help them make data-driven decisions. A key part of any modern business intelligence (BI) system are the reports that provide insights to drive change, pinpoint market opportunities and eliminate inefficiencies. Consequently, it is important for organizations to have a clear understanding of how all reports are managed, including how these reports are being used, how often, and by whom. Rationalization using data science can empower organizations to determine the right set of reports expeditiously.
Report rationalization provides significant benefits to those embarking on business intelligence transformation or consolidating reports arising out of mergers and acquisitions. For example, some 60% of organizations in the next three years plan to migrate or move BI/analytics workloads to the cloud, according to the 2020 IDG Cloud Computing Survey. Migrating a BI system (or multiple disparate BI systems) and often thousands of reports can slow the pace to a crawl. This can make the journey to modern BI more expensive and less efficient.
Unfortunately, most report rationalization processes are primarily manual in nature. In our experience, the typical manual process for report rationalization requires approximately 10 hours per report — very time consuming and cost prohibitive even for a relatively small set of 100 reports.
The need for a new approach
Clearly, businesses need an alternative solution to manual report rationalization, as well as a way to perform rationalization cost-effectively on a regular basis. Fortunately, a new approach has emerged that utilizes the promise of data science to reduce the cost, time and complexity of managing and consolidating reports. This white paper will explore how data science and artificial intelligence (AI) are being used as part of a new strategy to modernize report rationalization.
Overall, the reporting ecosystem in many organizations today can be described as a mashup of document formats within multiple, siloed BI systems, underlining the need for report rationalization. Large numbers and varieties of reports must be managed, and this task becomes more complex if reports are distributed across different lines of business, products, geographic locations, regions and countries.
Mergers and acquisitions pose yet another challenge of migrating a large volume of reports. Costs increase as IT requires a larger budget to keep up with increasing computing costs.
Cost reduction is another driver for any report rationalization effort. For instance, a major investment bank that used the Iris rationalization accelerator reduced its report volume of 4,000 by 25%, which helped it trim maintenance costs.
In our experience, it is estimated that it costs a business between $4,000 and $10,000 to build and manage a single report within a BI system over the life of the report. Now multiply those numbers by the hundreds of reports generated over the years to accommodate the deluge of BI data flowing into multiple disparate systems across the enterprise. Not only is this cost prohibitive, but unfortunately, maintaining a large volume of reports does not necessarily result in better business insights.
An intelligent approach to solving these challenges is to abandon manual processes and adopt a modern report rationalization strategy that leverages data science — specifically, natural language processing (NLP) and machine learning (ML) techniques.
Applying data science for report rationalization
A new approach to report rationalization is gaining traction where the tools and techniques of data science are applied to simplify and improve the benefits of report rationalization. Data science applies a variety of different methods, systems, processes and algorithms to both structured and unstructured data to glean valuable insights. ML is based around the use of algorithms that automatically improve through experience while NLP is primarily focused on using computers to process and analyze large amounts of natural language data.
Using NLP to extract and read report headers or data labels — not the data itself — in any document type and from any platform enables rationalization to be both BI-application and format agnostic. In addition, this new approach also leverages machine learning (ML) to provide a comprehensive review of all the existing reports and documents.
With ML it is possible to automatically identify duplicates (reports providing data on the same report fields) as well as similar reports (reports with an overlap of report fields) so that only the ones that are required by the business and used regularly will be consolidated. The machine learning component also helps data and business analysts in their efforts to standardize and define metadata.
Advantages of report rationalization with data science
Data science-driven report rationalization is platform agnostic and is beneficial to organizations across industries. The key benefits of this solution are:
Cost reduction of 25-40%: Report rationalization driven by data science has consistently delivered more than a 30% reduction in the total volume of reports. In our experience, the volume of reports has been reduced by over 70% and costs by up to 40% in some cases. Industry estimates indicate that the savings from even a 10% reduction of reports will pay for the cost of the rationalization effort itself.
More insights from fewer reports: BI consolidation can provide better insights from a smaller, more efficient report set. This makes it easier to identify duplicates, and identify any redundancies — as well as significantly reduce maintenance costs.
Supports BI modernization: Additionally, the results of a data science-driven report rationalization solution can be leveraged in the execution of a BI transformation initiative aimed at migrating reports from a traditional BI platform to a modern one. After rationalization, the optimal number of reports can be migrated to the new system.
Key takeaways
- For organizations seeking to reduce report volume and costs, or undergoing a BI transformation, report rationalization driven by data science makes it easier, faster and less expensive.
- When applied to BI consolidation and transformation initiatives, report rationalization typically reduces the total number of reports by approximately 30%.
- When driven by data science, rationalization recommendations can be obtained in a matter of weeks.
- Often considered an afterthought, report rationalization should be performed upfront in any BI modernization or transformation effort.
- Whether part of a larger initiative or as a standalone process, now is the time for businesses to take advantage of emerging trends in NLP, machine learning and AI by adopting a faster, proven, report rationalization solution that is driven by data science.
Explore more on our solutions and services in Data & Analytics.
Contact
Our experts can help you find the right solutions to meet your needs.
Get in touch