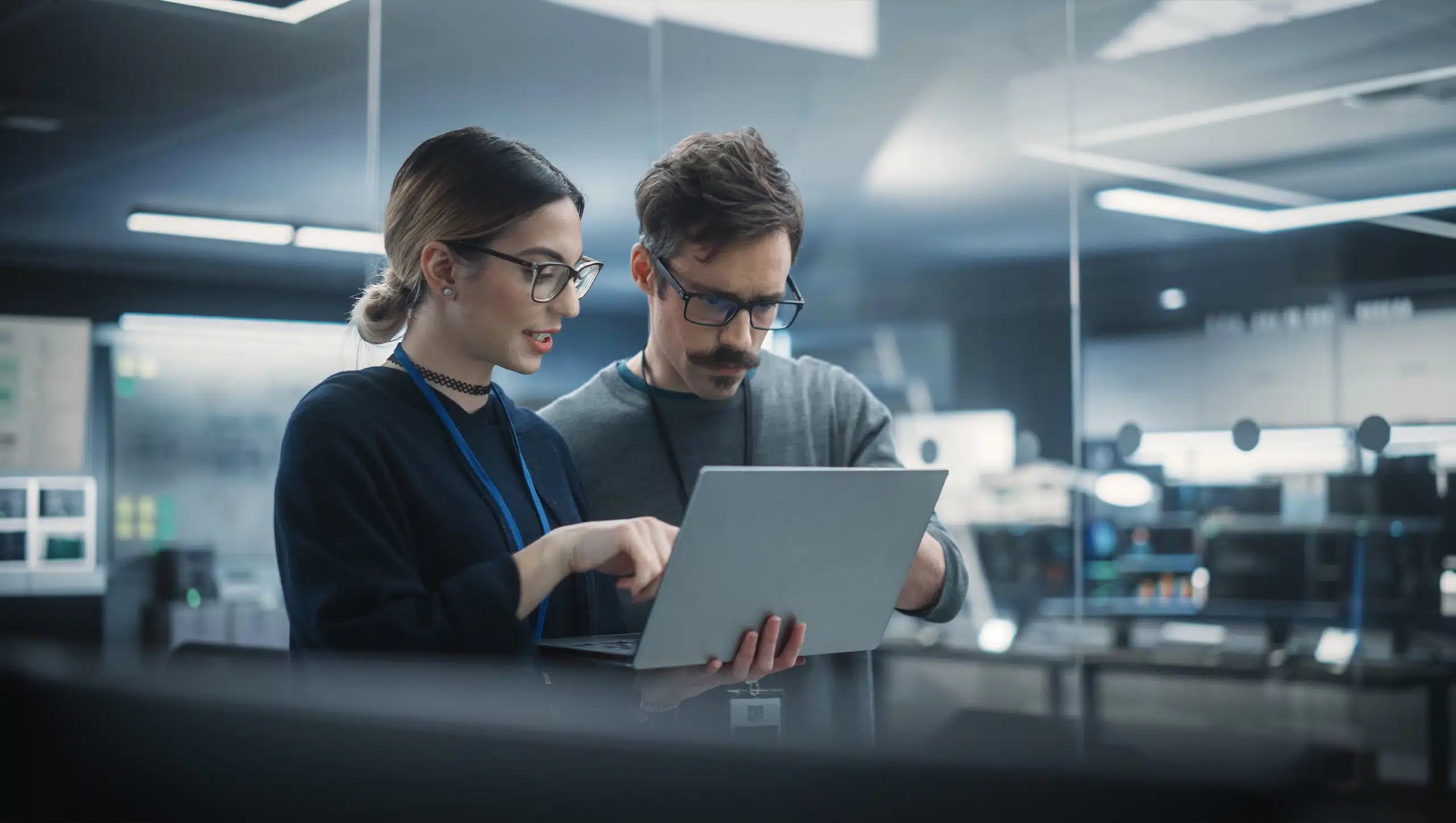
Productionizing Generative AI Pilots
Get scalable solutions and unlock insights from information siloed across an enterprise by automating data extraction, streamlining workflows, and leveraging models.

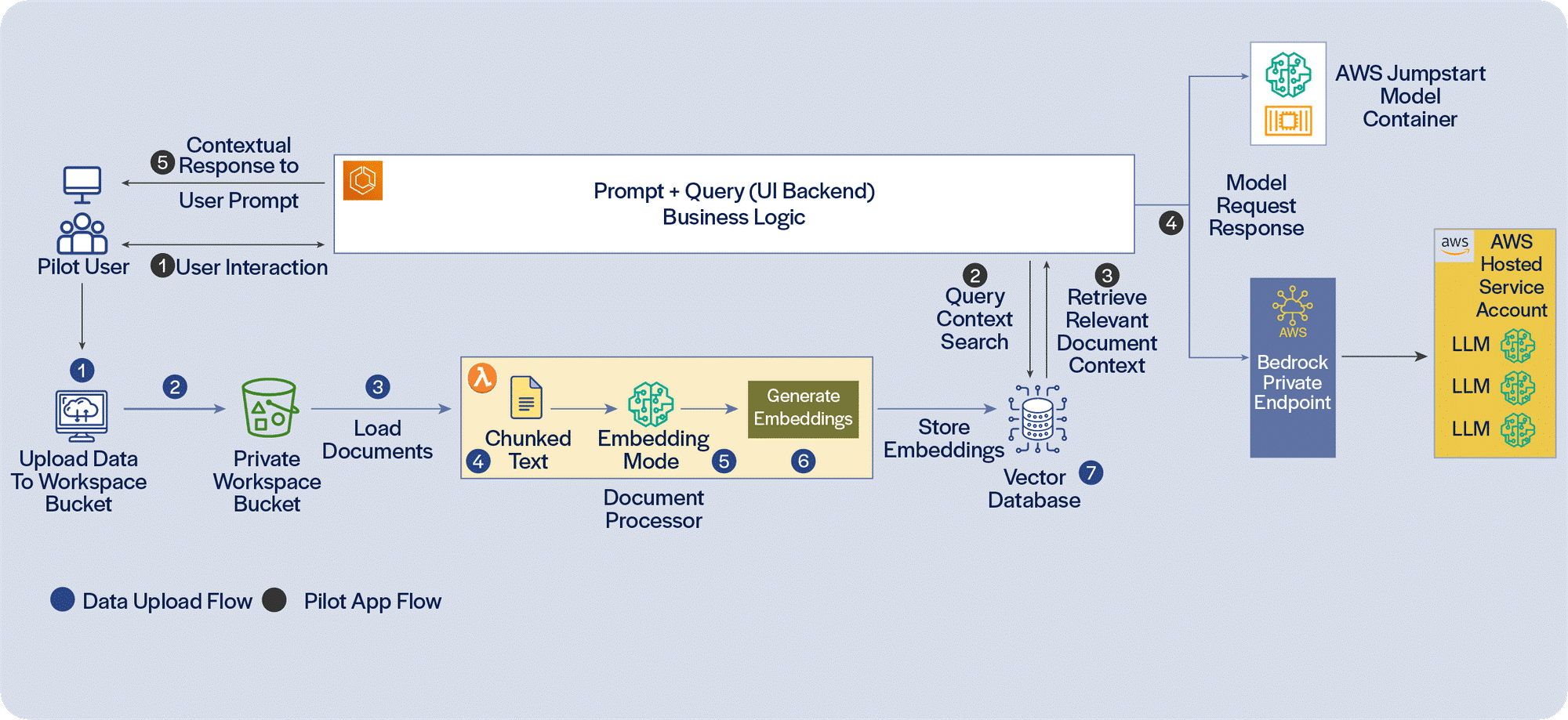
Enterprises have vast amounts of unstructured information such as onboarding documents, contracts, financial statements, customer interaction records, confluence pages, etc., with valuable information siloed across formats and systems.
Generative AI is now starting to unlock new capabilities, with vector databases and Large Language Models (LLMs) tapping into unstructured information using natural language, enabling faster insight generation and decision-making. The advent of LLMs, exemplified by the publicly-available ChatGPT, has been a game-changer for information retrieval and contextual question answering. As LLMs evolve, they’re not just limited to text. They’re becoming multi-modal, capable of interpreting charts and images. With a large number of offerings, it is very easy to develop Proofs of Concept (PoCs) and pilot applications. However, to derive meaningful value, the PoCs and pilots need to be productionized and delivered in significant scale.
PoCs/pilots deal with only the tip of the iceberg. Productionizing needs to address a lot more that does not readily meet the eye. To scale extraction and indexing information, we need to establish a pipeline that, ideally, would be driven by events, new documents generated and available, possibly through an S3 document store and SQS (Simple Queue Service), to initiate parsing of documents for metadata, chunking, creating vector embedding and persisting metadata and vector embedding to suitable persistence stores. There is a need for logging and exception-handling, notification and automated retries when the pipeline encounters issues.
While developing pilot applications using Generative AI is easy, teams need to carefully work through a number of additional considerations to take these applications to production, scale the volume of documents and the user-base, and deliver full value. It would be easier to do this across multiple RAG (Retrieval-Augmented Generation) applications, utilizing conventional NLP (Natural Language Processing) and classification techniques to direct user requests to different RAG pipelines for different queries. Implementing the capabilities required around productionizing Generative AI applications using LLMs in a phased manner will ensure that value can be scaled as the overall solution architecture and infrastructure is enhanced.
Read our perspective paper for more insights on Productionizing Generative AI Pilots.
Contact
Our experts can help you find the right solutions to meet your needs.
Get in touch