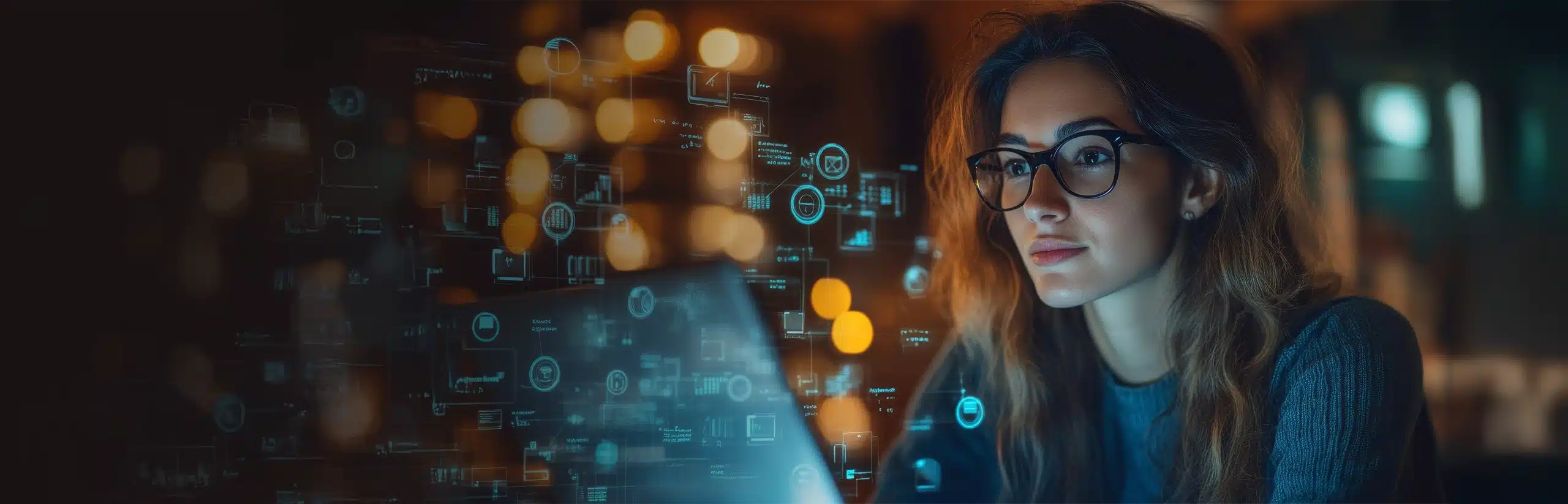
Commercial & Corporate Banking
Gen AI platform offers future-ready capabilities

Client
A leading North American bank
Goal
Provide a wide range of AI capabilities for various risk and business teams and avoid building fragmented, outdated systems
Tools and Technologies
Amazon Bedrock and Titan V2, pgvector, Faiss, OpenSearch, Llama 7B, Claude Sonnet 3.5 and 3.7
Business Challenge
The Enterprise Risk function at a leading North American bank initiated a Generative AI (Gen AI) solution to offer a wide range of AI capabilities, including document intelligence, summarization, generation, translation, and more.
As the project evolved through proofs of concept and pilots, a key challenge emerged: the risk of creating a fragmented ecosystem with an overwhelming array of unmanageable bespoke solutions, model integrations, and reliance on potentially outdated models and libraries.

Solution
Based on prior engagements across clients, our team delivered thought leadership around how to develop and deliver capabilities using a platform approach. We also set up a Minimum Viable Product Team to iterate on new problem areas and solution approaches. Platform development includes generalized capabilities for:
- Setting up document ingestion pipelines, with choice of parsing approaches, embedding models and vector index stores
- A factory model along with configurations for integrating new parsers, embedding models, LLM interfaces etc., to quickly bring new capabilities to the platform
- User management, SSO integration, entitlements management
- API integration to bring in information/ data from internal and external sources
- Platform support of pgvector, Faiss, OpenSearch, Amazon Titan V2, Llama 7B, Claude Sonnet 3.5, and 3.7, etc.
- Intuitive chat interface for AI Masters - designated business users trained in Prompt Engineering and other techniques to assemble new AI/Gen AI capabilities for users through configuration - and end users

Outcomes
- A future-ready Gen AI platform that can easily incorporate new capabilities and updates
- Multiple specific capabilities, called skills, for use by various risk teams and business users
- A forward-looking roadmap, including ability to compose more complex capabilities using atomic capabilities

Our experts can help you find the right solutions to meet your needs.
Join us at Reuters Pharma USA 2025
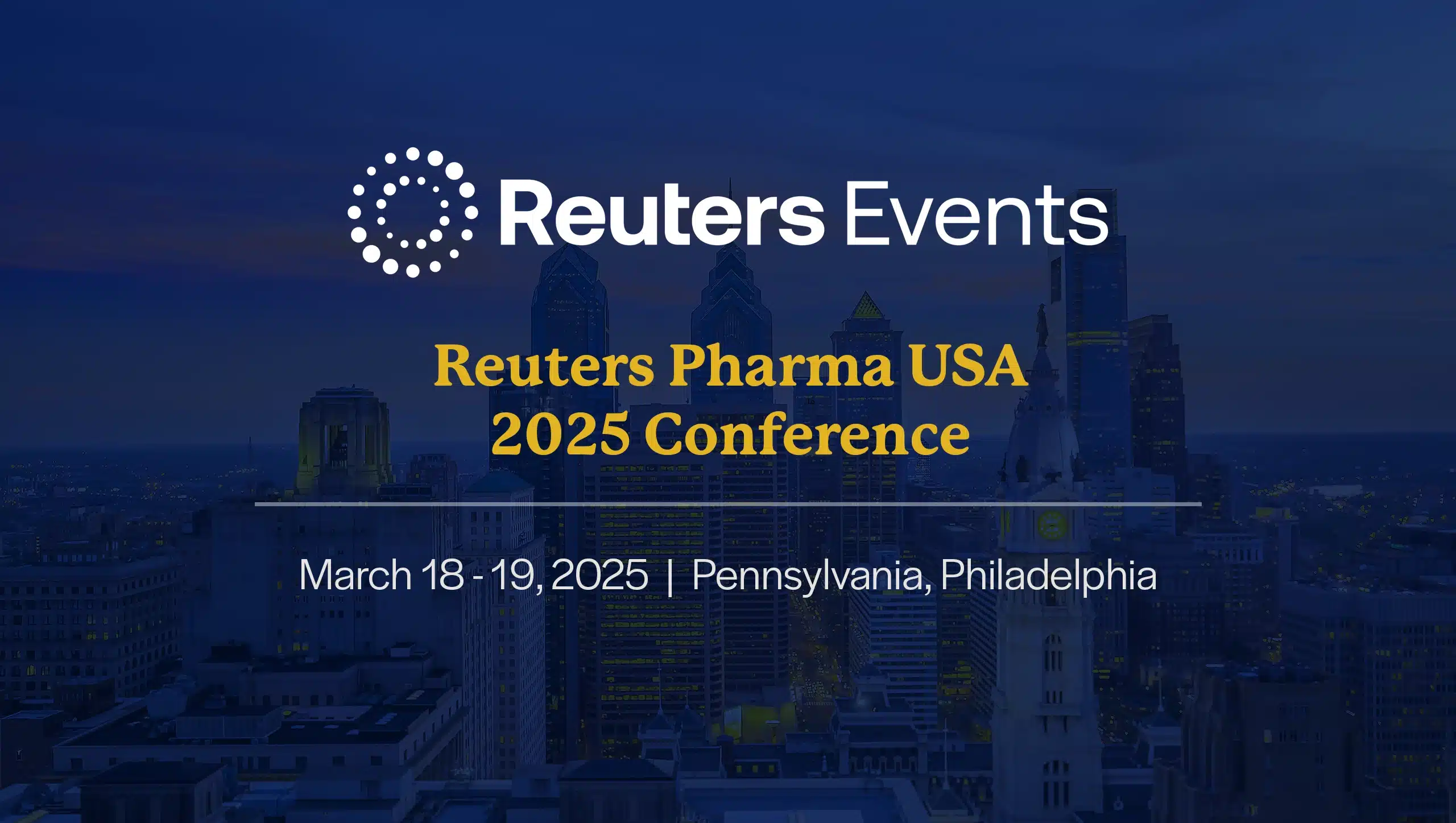
Reuters Pharma USA 2025 Conference, noted as North America’s largest cross-functional pharmaceutical gathering, is scheduled between March 18-19, 2025, at the Pennsylvania Convention Center in Philadelphia. With the pharmaceutical industry continually pressured by shifts in market trends and participants, product development, consumer sentiment, and government regulations, attendees and speakers at this Conference will be seeking and sharing insights and strategies to best navigate change, remain competitive, and future-proof operational units as well as global enterprises.
Swarnendu Banerjee, client partner and seasoned IT professional for the pharmaceutical and life sciences sectors, will be attending the Reuters Pharma USA 2025 Conference. He will share Iris’ extensive experience in these domains. How our advanced capabilities in AI/Generative AI, Application Development, Intelligent Automation, Cloud, Data & Analytics, Integrations, and Quality Engineering have delivered successful outcomes in mission-critical engagements, ensuring quality and compliance, reducing costs, enhancing UX, modernizing, migrating, scaling, accelerating, and streamlining.
Connect with Swarnendu at the Reuters Pharma forum or anytime, or visit our Services and Life Sciences capabilities pages to explore our innovative approach and strategies for end-to-end digital transformation.
Contact
Our experts can help you find the right solutions to meet your needs.
Get in touchTransforming payment processing for banking channels
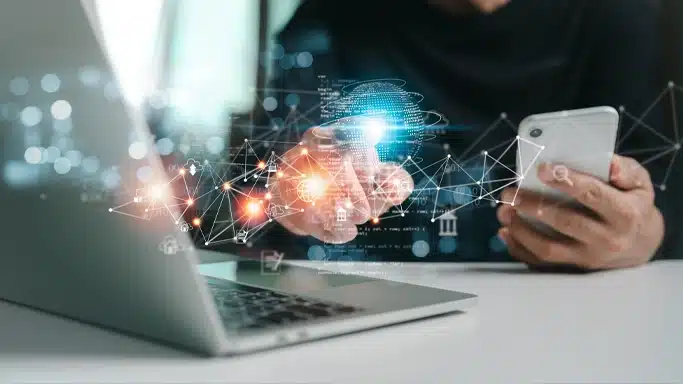
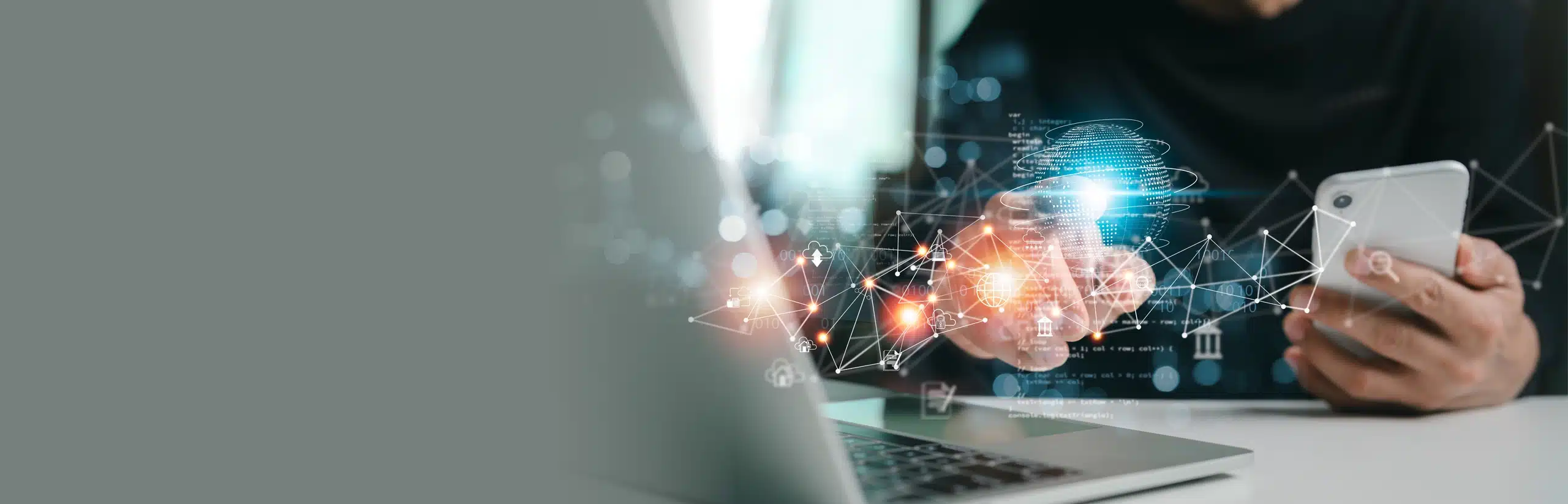

Client
A leading Australian bank
Goal
Streamline the payment transaction lifecycle to handle increasing volume and complexity
Tools and Technologies
Jenkins, Kubernetes, Spring, Oracle, PostgresSQL, AWS, Docker, Kafka, Java
Business Challenge
Multiple banking channels - mobile, internet and branch - initiate various payment requests (20+ types such as ACH, mandate and book transfer) that need to be processed. Depending on the payment type, the channel was required to invoke one or more services in a specific order as per the associated business rules.
Changes to these payment workflows stemming from introduction of new payment types or revisions of business rules introduced complex and repeated changes to the bank’s systems, hindering scalability.

Solution
- To support lifecycle management of various payment transactions, including defining different payment workflows, we designed an event-driven architecture comprised of 40+ microservices (e.g., limit, eligibility, and fraud checks, etc.) supported by a Kafka message queuing system
- The architecture involved building an orchestration engine (landing service), acting as a front controller for all payment workflow requests from the various banking channels, such as mobile, internet and branch
- The landing service in turn invokes the corresponding service (limit, eligibility, etc.) based on the payment type and business rules associated with it
- Data flow between these microservices (resulting from further invocations) and other downstream systems is facilitated asynchronously with the help of a distributed messaging system (Kafka)
- Using Jenkins, we built a CI/CD pipeline to streamline the workflow by automatically building, testing and deploying code changes as they are committed

Outcomes
- Significantly eased the management of payment workflows, including those related to the addition of new payment types (resulting from an acquisition)
- Enabled systems to scale without introducing complex changes at the channels
- Improved reporting, resulting from faster access to data through dedicated microservices

Our experts can help you find the right solutions to meet your needs.
Seamless 24/7 Operations with App Management
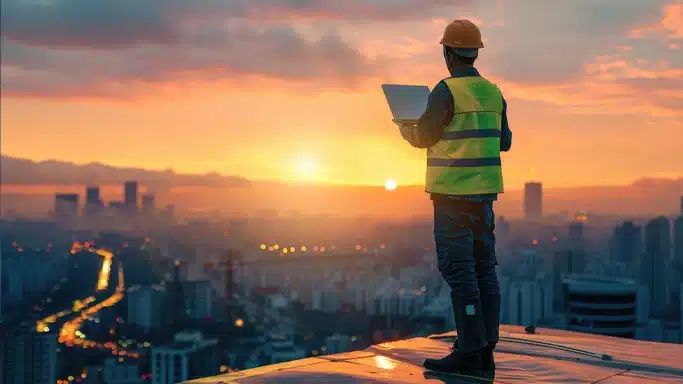
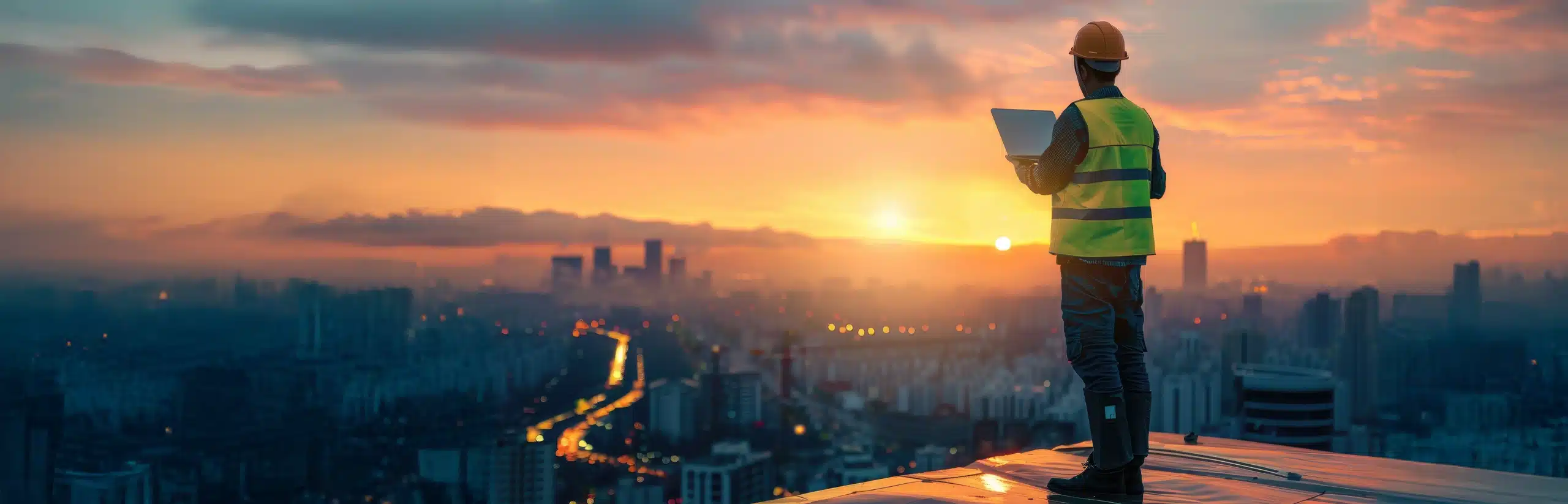

Client
A leading manufacturer of roofing materials
Goal
Achieve seamless, 24/7 operations with >95% SLA compliance while efficiently managing diverse technology platforms.
Tools and Technologies
.NET Azure applications and services, Sitecore, MuleSoft, Google Cloud Platform (GCP), PeopleSoft, Salesforce
Business Challenge
Ensuring uninterrupted 24/7 business operations involves supporting and maintaining critical applications across diverse technology stacks. This includes achieving near-perfect Service Level Agreement (SLA) compliance by consistently delivering response and service levels exceeding 95%.
Managing a complex technology ecosystem spanning platforms like .NET, Azure, Salesforce, Sitecore, MuleSoft, GCP, and PeopleSoft, requires expertise and coordination. Additionally, the roofing industry's seasonal nature and frequent deployments lead to unforeseen spikes in incidents and requests, demanding dynamic resource allocation and effective load-management strategies to efficiently meet unpredictable demand.

Solution
- Cross-training of an agile team on all platforms, enabling them to handle spikes without SLA impact, shifting focus dynamically
- Proactive use of monitoring tools and automation, which reduced incidents and resolution time by addressing issues early
- A Gen AI-powered knowledge base documented resolutions, enabling faster, accurate incident handling
- Ensured seamless communication across platform systems, like Salesforce and PeopleSoft, for quick integration fixes
- Regular customer feedback loops and monthly reports aligned system performance with business needs, highlighting insights and improvements

Outcomes
- Near-perfect SLA adherence at 99.9%, ensuring minimal business disruption on an ongoing basis
- Managed 1,100+ incidents per month with a decrease in resolution times stemming from proactive monitoring and streamlined processes
- 25% uptime improvement from proactive maintenance, ensuring high application availability and minimal downtown
- 24/7 load handling that effectively managed spikes during demand surges, maintaining SLAs without extra resources
- Continuous optimization from regular RCAs (Root Cause Analyses) and process enhancements, which reduced incidents and improved system efficiency

Our experts can help you find the right solutions to meet your needs.
Modernized Payments Hub Improves UX and Compliance
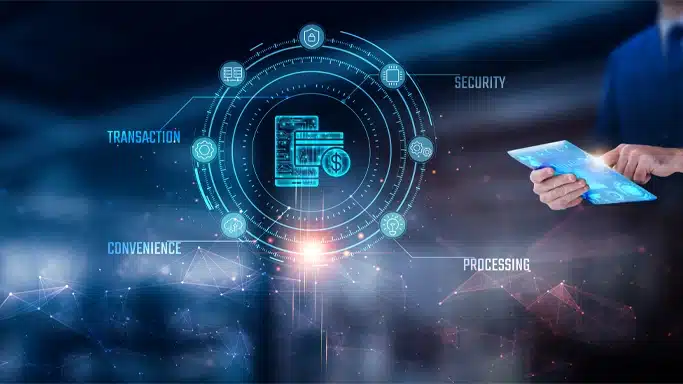
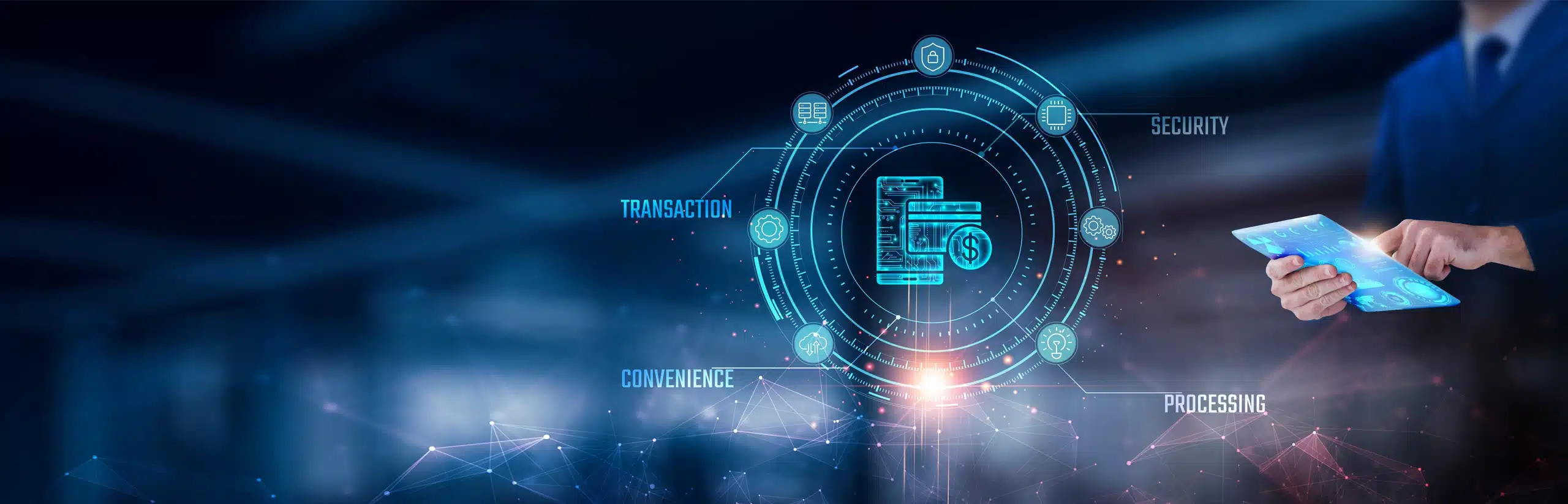

Client
U.S. operations of a leading Japanese bank
Goal
Modernize payments architecture to streamline processing and improve client experience
Tools and Technologies
Jenkins, Kafka, Spring, Oracle, JBoss, React, Elastic Search, Java, Node.js
Business Challenge
The evolving payments landscape, with the introduction of ISO 20022 and the dynamic nature of the regulatory environment, necessitated advancement in the bank’s payment processing capabilities.
The lack of a modern architecture hindered client experience, with multiple channels initiating various payment types that required complex processing.

Solution
Our team built a centralized payments hub to orchestrate data flows between payment initiation systems and product processors. The steps:
- Designed a flexible and scalable microservices-based architecture to facilitate translation, enrichment and processing of payment transactions
- Built a messaging layer to streamline data flows between systems, through support for various modes of interaction, e.g., MQ, API and file (canonical / industry standards such as NACHA, SWIFT, JSON, etc.)
- Introduced an API gateway to handle multiple payment types to enable channel agnostic payment capabilities
- Deployed a modular approach to support existing and new systems with isolation of core and product processors and avoid redundancies in capability builds
- Developed a React-based UI as the touchpoint for integrations between the payments hub and other systems

Outcomes
- A core payments engine capable of seamlessly integrating with multiple, complex systems
- Superior client experience, resulting from a holistic view spanning initiation, payment rails, and clearing
- A modernized payments platform that is ISO 20022-compliant and future-ready for processing and reporting needs
- Faster implementation of functionalities for payment processors

Our experts can help you find the right solutions to meet your needs.
New IT ecosystem earns 100% satisfaction rating
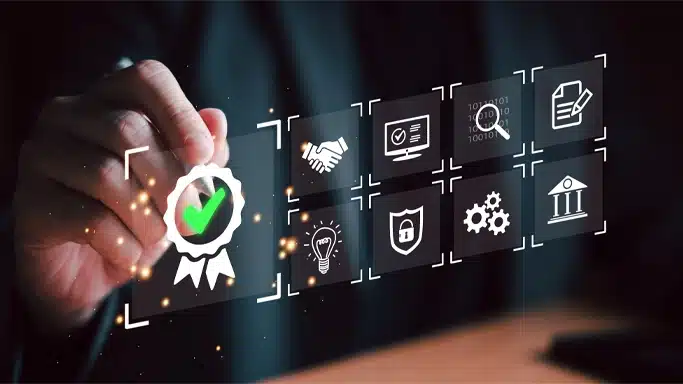
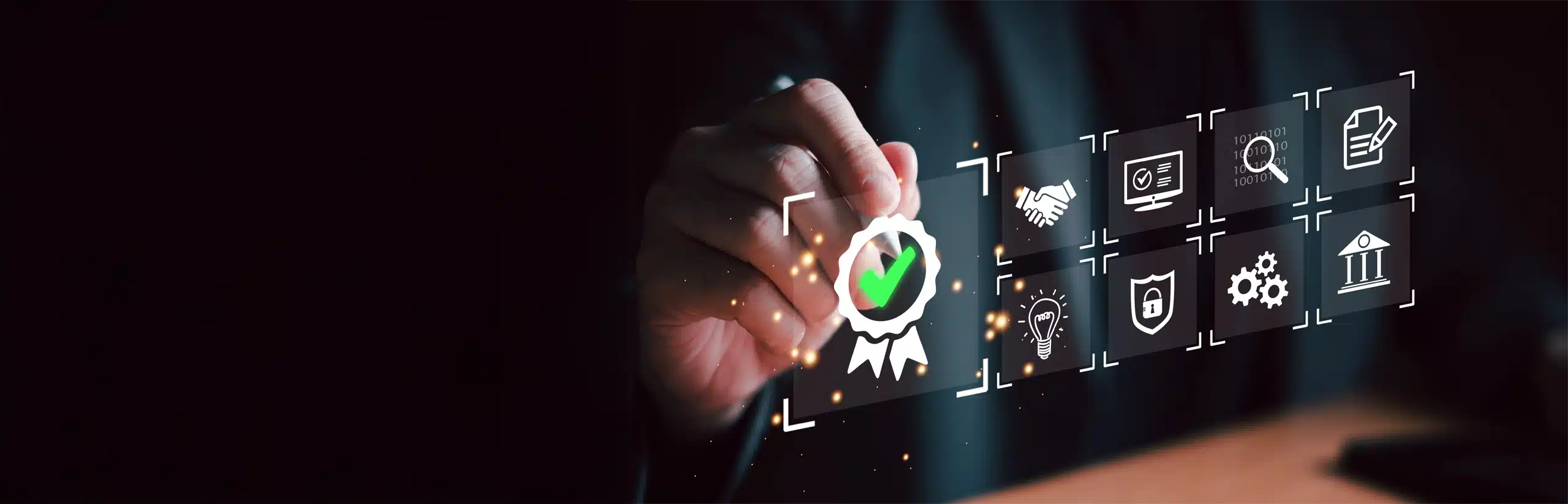

Client
A leading global standards organization
Goal
Improve coordination across teams, reduce delivery delays, and streamline support processes for global users
Tools and Technologies
.NET Core, Vue.JS, Python, Docker, Kubernetes, Azure, Angular, Cosmos DB, MS SQL Server, PowerBI, Redis, Azure Functions, Azure Data Factory, Azure App Service, Spring Boot, Java
Business Challenge
The IT ecosystem was spread across multiple vendors and in-house teams, creating significant coordination overheads and challenges. These issues led to member organizations expressing dissatisfaction due to delivery delays and high turnaround times on incidents and problems.
The teams also had to support users across various geographies and time zones, further complicating operations. A lack of standardized customer service processes and knowledge base documentation also hindered issue resolution. Additionally, the teams struggled with awareness of client-specific standards and applications, while needing to handle ad hoc requests and support teams with customer service-specific projects.

Solution
- Three-week discovery phase and six-month transition plan covering 10+ applications, 24/7 Level 2 support, and infra support
- Established service and operations management processes, including governance, tools, and KPIs for agile and ITIL processes
- Set up and maintained Freshdesk ticketing system to manage user requests
- Created detailed SOPs and canned messages in Freshdesk for BAU tracking
- SLA collaboration - worked with L3 team to define resolution SLAs for critical tickets
- Created weekly and monthly reports to track requests, issues, and risks
- Set up a standard process with client to handle access-related requests

Outcomes
- Zero downtime deployments
- Faster on-boarding of member organizations
- Continuous reductions in infra costs on quarter-to-quarter basis
- 100% response and resolution SLA that led to 100% customer satisfaction
- Timely access to all applications for business users
- A one-stop shop via Freshdesk for all ticket information and data for all stakeholders
- Expertise on global standards enables ideas and suggestions to optimize the process for continuous improvement

Our experts can help you find the right solutions to meet your needs.
Automated Scheduling Bots Boost Productivity by 50%
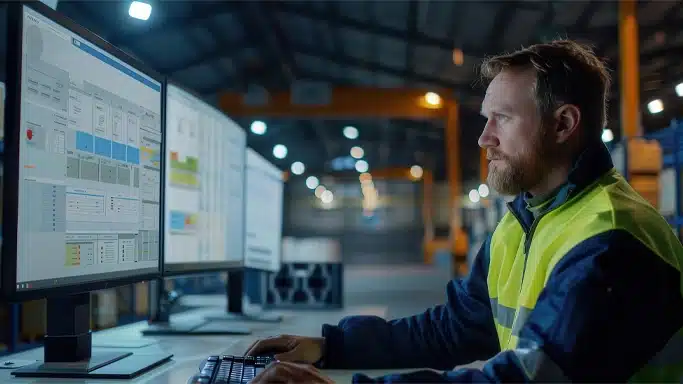
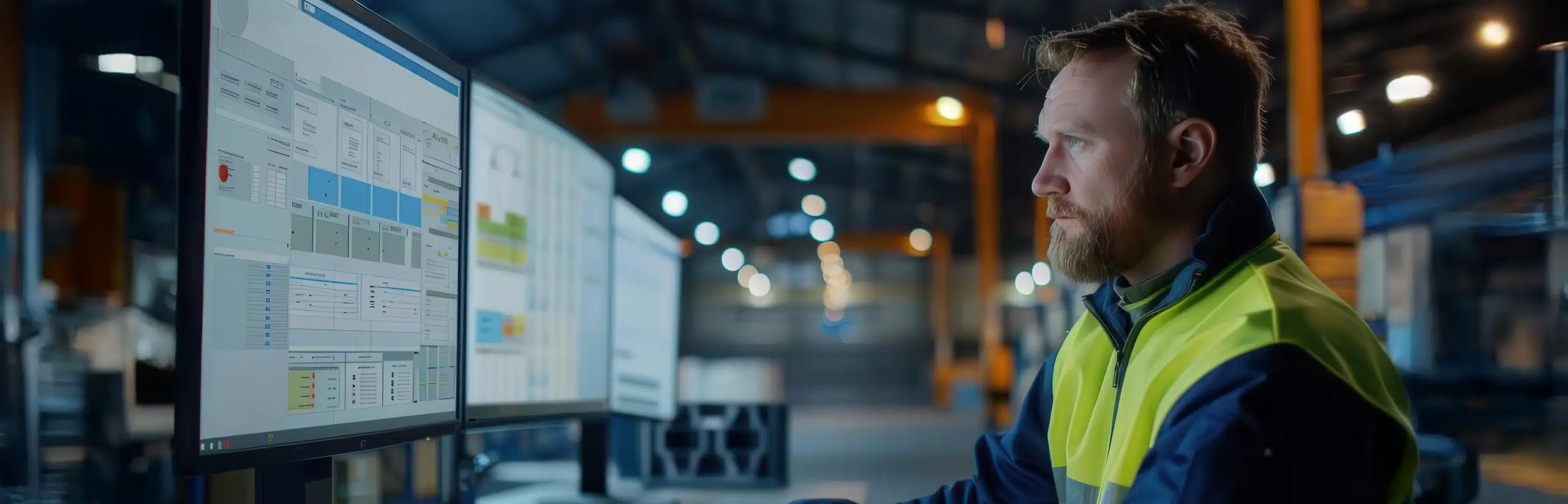

Client
Leading supply chain brokerage
Goal
Automate the manual, supply-chain scheduling process to improve staff productivity and customer satisfaction
Tools and Technologies
UI Path Orchestrator, UI Path Assistant, Microsoft Power BI, Office 365
Business Challenge
Performing crucial supply chain logistics, a provider’s operations team was struggling due to the high volume of scheduling appointments with shippers, receivers, and carriers, which involve back and forth emails, phone calls, or manual data entry into multiple Transport Management Systems (TMS).
These appointment-scheduling complexities vary based on the parties involved, from sending an email requesting appointment times to accessing a TMS and selecting what’s available as per their schedule.
Lacking proper analytics, sales representatives were unable to pinpoint peak appointment times, track cancellation rates, or discern customer preferences, often leading to shipment delays and incurred detention charges.

Solution
- Deployed multiple rule-based, automated workflows to pull information from incoming appointment requests (from emails, web forms, etc.) and automatically input it into the various TMS used to book pick-up and delivery appointments
- Developed a Power BI dashboard to visualize appointment trends, peak times, and cancellation rates, providing insights into customer behaviors, including frequent reschedules, preferred times, and typical lead times for booking appointments
- Delivered a reusable solution that could be leveraged for other business areas

Outcomes
- Bots operating 24/7 have led to over 15,000 monthly appointments being scheduled, resulting in a 50% reduction in manual scheduling hours
- The productivity of the operations team has improved by 50%, enabling staff to concentrate on high-value tasks rather than manual appointment-booking
- The increased accuracy in scheduled appointments has significantly decreased detention charges, thereby boosting overall customer satisfaction

Our experts can help you find the right solutions to meet your needs.
Automated POD improves turnaround time 95%
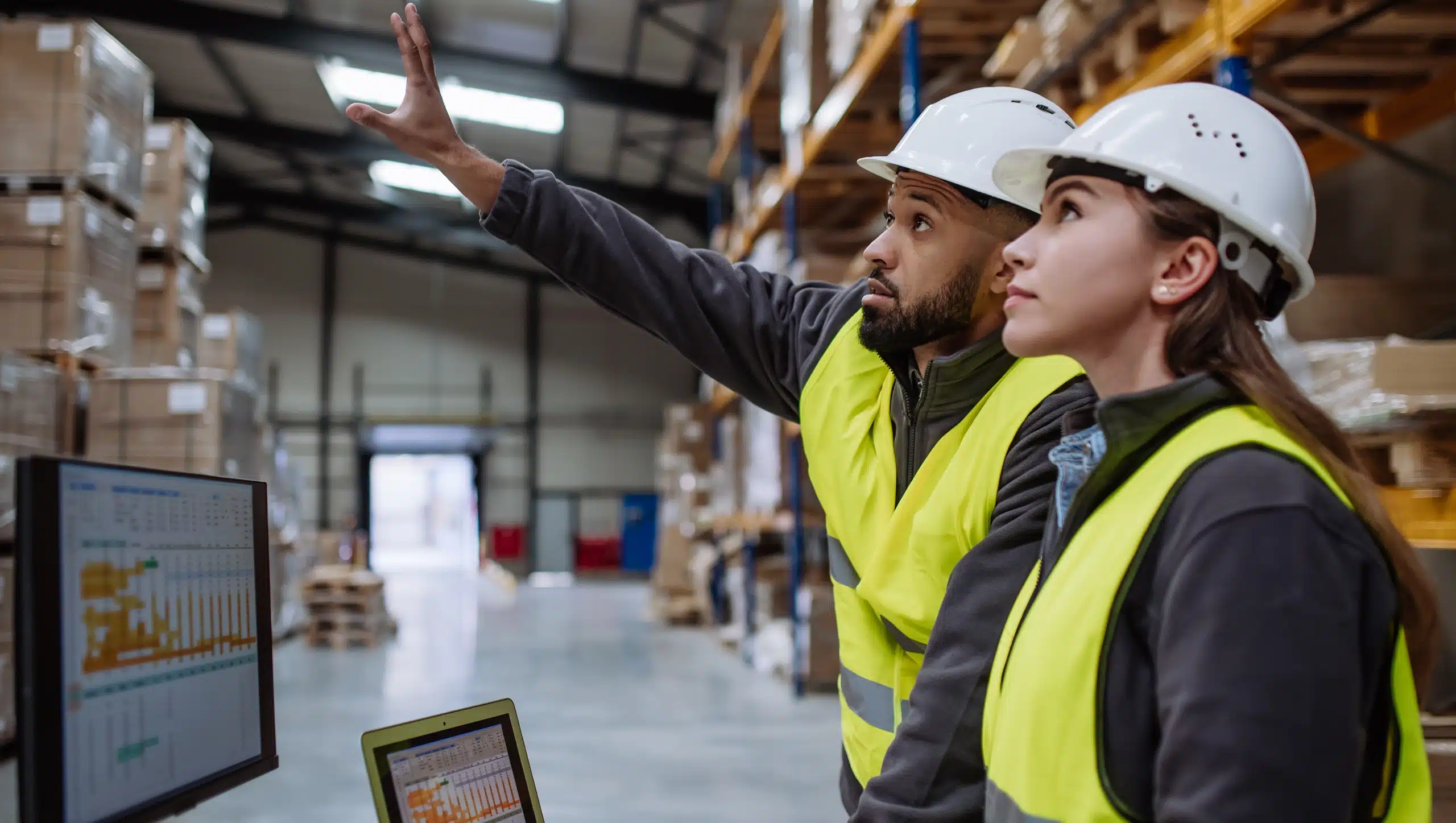
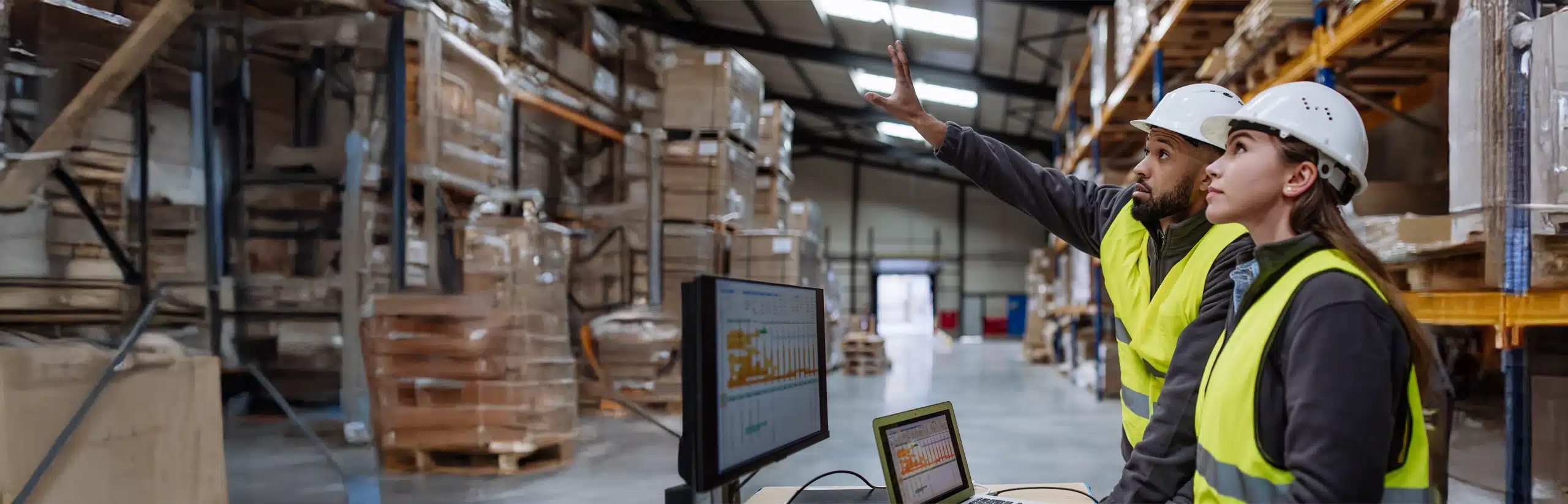

Client
Leading supply chain brokerage
Goal
Automate Proof of Delivery documentation process to increase efficiency and accuracy in data upload, validation and invoicing
Tools and Technologies
UI Path Orchestrator, UI Path Document Understanding, Microsoft Power BI, Oracle Transportation Management
Business Challenge
Proof of Delivery (POD) is a document that confirms an order has arrived at its destination and was successfully delivered before the invoice can be billed for payment.
Lack of an electronic POD system leads to inefficient, manual processing due to varied legal and contractual documentation requirements, resulting in longer billing cycles. Diverse formats and layouts from different carriers complicate data extraction from paper-based PODs.

Solution
- Developed a Document Processing Bot with UI Path AI Center, leveraging Document Understanding and Optical Character Recognition for managing various carrier documents
- Optimized data models for major carriers, focusing on the top five document types that represent 80% of the volume
- Implemented UI Path Action Center's "Human in the Loop" to handle exceptions and conducted 6-8 weeks of rigorous training on the Document Understanding model to ensure accuracy and meet confidence targets

Outcomes
- Achieved a 95% reduction in POD turnaround time, dropping from 48 hours to 2 hours, significantly boosting customer satisfaction
- Enhanced productivity by 87.5%, confirming receipt and condition of freight efficiently
- Reached 80% process accuracy, with continuous enhancement via automatic retraining
- Cut the billing cycle by 35%, allowing immediate use of data for customer invoicing

Our experts can help you find the right solutions to meet your needs.
Unified automation strategy enhances efficiency
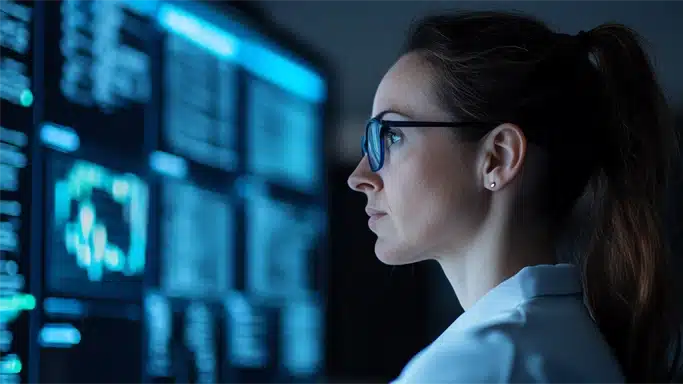
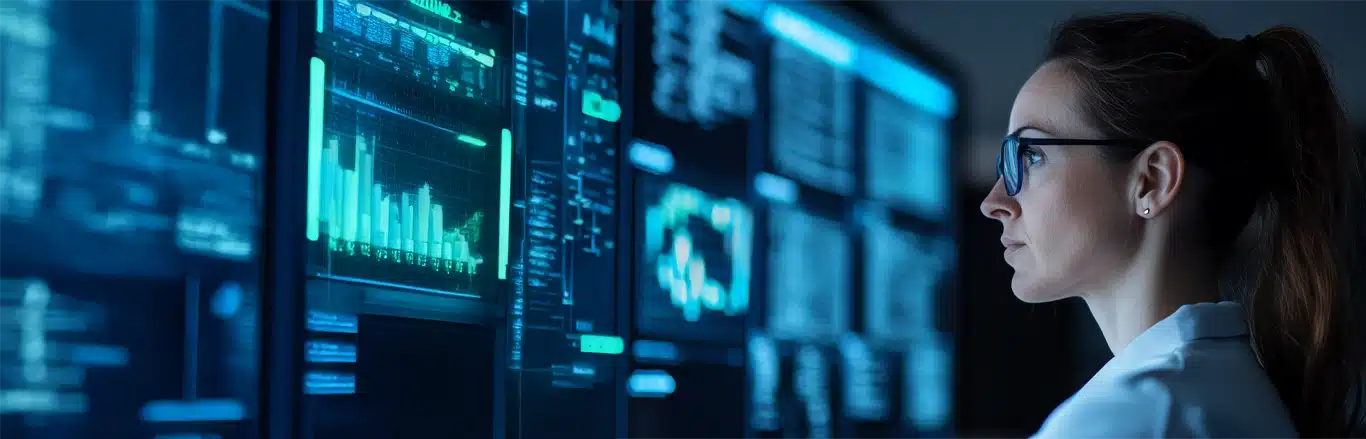

Client
Leading payroll and HR solutions provider
Goal
Develop automation strategy and framework that accommodates growth and ensures efficiency
Tools and Technologies
Ansible, AWS, Dynatrace, Gremlin, Groovy, Jenkins, Keptn, KICS, Python, Terraform
Business Challenge
The SRE (Site Reliability Engineering) shared services team faced a diverse set of needs relating to automation of infrastructure and services provisioning, configuration, and deployment.
The team was encountering resource constraints, as limited in-house expertise in certain automation tools and technologies was causing delays in meeting critical automation requirements. They also needed to ensure system reliability and were challenged to scale automation solutions to accommodate increasing demands as operations grow.

Solution
- Development of a comprehensive automation strategy to align with objectives, encompassing Terraform, Ansible, Python, Groovy, and other relevant technologies in the AWS environment
- Leveraging our expertise to bridge the knowledge gap, provide training, and augment the client team in handling complex automation tasks
- Implementation of a chaos engineering framework using Gremlin, Dynatrace, Keptn, and EDA tools, to proactively identify weaknesses and enhance system resilience
- Creation of a scalable automation framework that accommodates growing needs and ensures long-term efficiency

Outcomes
- A unified automation strategy that streamlined processes, reduced manual effort, and enhanced overall efficiency by 30%
- The implementation of chaos engineering and self-healing practices, which increased reliability between 20% and 50%
- A reduction in manual interventions along with improved efficiency that will result in cost savings of 25% - 50%

Our experts can help you find the right solutions to meet your needs.
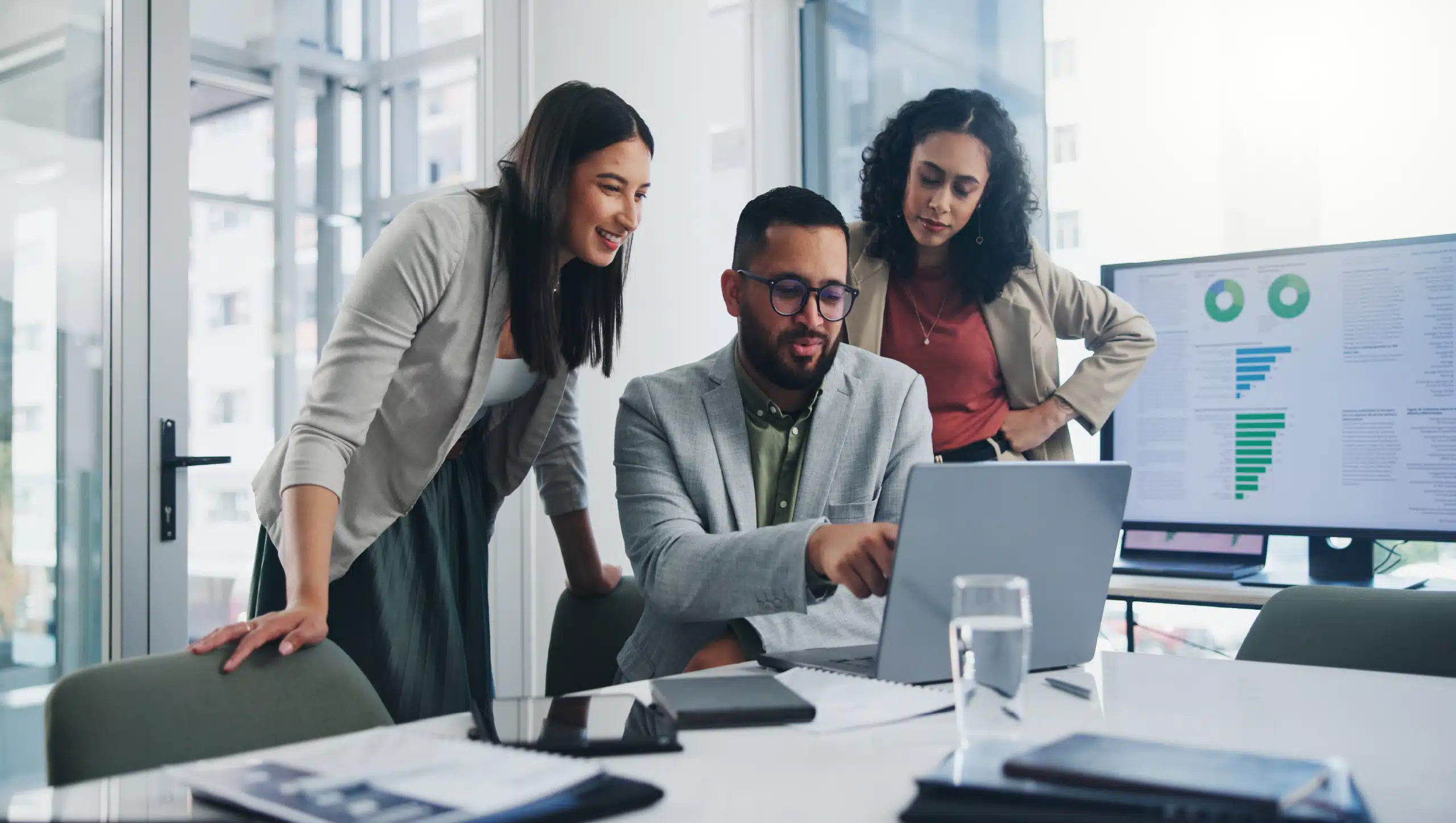
The evolution of quality engineering
Quality engineering in software development empowers organizations to achieve heightened quality, scalability and resilience.

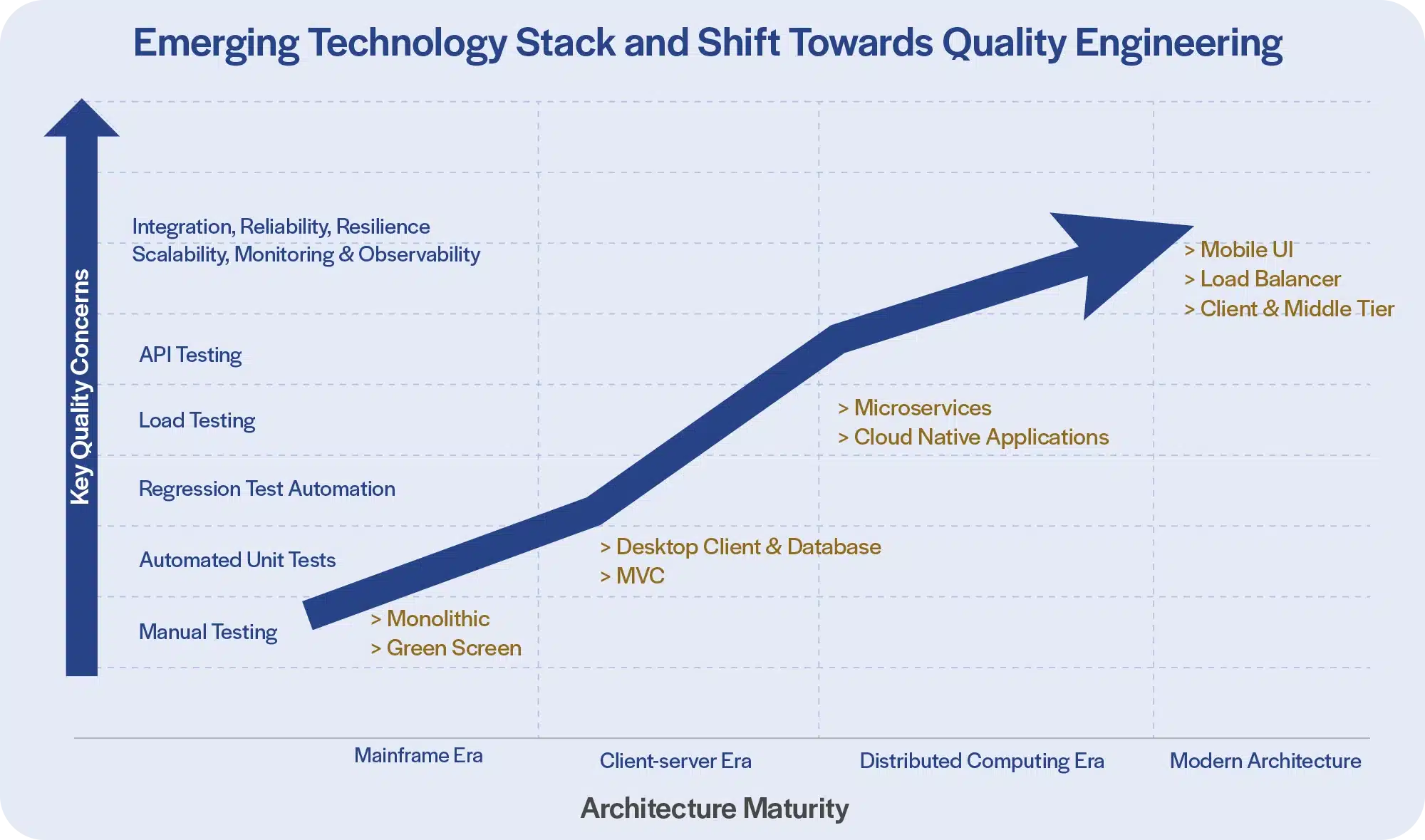
Quality Assurance (QA) has long been essential in software engineering, ensuring the development of products and applications with established standards and metrics. But QA has been reactive, focusing on defect detection through manual and automated testing. With the evolution of software development technology and methodologies, the limitations of traditional QA are evident. This perspective paper delves into the evolution of Quality Engineering (QE), which has transformed the approach to software quality. QE goes beyond QA and Test Automation to integrate quality practices throughout the Software Development Lifecycle (SDLC); it also addresses complexities in modern architectures such as microservices and cloud environments.
The journey from QA to QE is marked by several key milestones. Initially, software testing was a separate phase, conducted after development was complete. With the advent of Agile and DevOps methodologies, the need for continuous testing and early defect detection became apparent. This shift fostered the evolution of testing practices, embedding quality checks throughout the development cycle with the emerging adoption of cloud-native modern architecture, paving the way for what is now called Quality Engineering. Unlike the reactive nature of traditional QA and QA Automation, QE represents a proactive and integrated approach throughout the development lifecycle.
Organizations can significantly enhance product or application quality, optimize development workflows, and mitigate risks by addressing QE concerns at every phase of the SDLC. They could leverage structured QE approaches as mentioned above, and focus on a holistic view of quality in modern architecture.
Read our Perspective Paper for more insights on the evolution of quality engineering in software development.
Contact
Our experts can help you find the right solutions to meet your needs.
Get in touchIndustries
Company
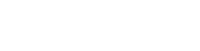